Comprendiendo la adopción de ChatGPT en universidades: el impacto del TPACK y UTAUT2 en los docentes
DOI:
https://doi.org/10.5944/ried.28.1.41498Palabras clave:
Inteligencia Artificial, TPACK, ChatGPT, modelo UTAUT2, instructoresResumen
El objetivo de la tecnología de inteligencia artificial (IA) es crear dispositivos inteligentes que realicen tareas que tradicionalmente han requerido inteligencia humana. ChatGPT es un programa basado en IA que proporciona instructores virtuales y un entorno de aprendizaje personalizado para los estudiantes. Eleva el estándar para los mejores intérpretes al presentar información de vanguardia y fomentar el desarrollo intelectual. Este estudio investigó la importancia del Conocimiento Pedagógico Tecnológico del Contenido (TPACK) de los instructores para determinar la intención de usar ChatGPT a la luz del modelo de la Teoría Unificada de Aceptación y Uso de Tecnología 2 (UTAUT2). La metodología fue un enfoque cuantitativo y los datos se recopilaron de 569 instructores en universidades saudíes. Los datos fueron analizados mediante análisis de rutas y Smart PLS. Los resultados mostraron que la Expectativa de Esfuerzo, la Influencia Social, la Motivación Hedónica y la Calidad de la Información no influyeron significativamente en la Intención de Comportamiento. Sin embargo, la Condición Facilitadora, el Valor de Aprendizaje (negativamente) y el Riesgo de Privacidad sí tuvieron efectos significativos en la Intención de Comportamiento. Además, el TPACK de los instructores tuvo un papel moderador significativo en la relación entre el Riesgo de Privacidad y la Intención de Comportamiento. Los resultados destacan la necesidad de mejorar el TPACK de los instructores con programas de desarrollo profesional para fomentar una intención positiva de usar ChatGPT en las universidades saudíes. Se recomienda a las universidades proporcionar suficiente apoyo y recursos para que los instructores adopten la nueva tecnología en su enseñanza.
Descargas
Citas
Alghatrifi, I. y Khalid, H. (2019). A systematic review of UTAUT and UTAUT2 as a baseline framework of information system research in adopting new technology: a case study of IPV6 adoption. 6th International Conference on Research and Innovation in Information Systems. IEEE. https://doi.org/10.1109/ICRIIS48246.2019.9073292
Al-Safadi, H. A., Shgair, M. S. A. y Al Qatawnih, K. S. (2023). The Effectiveness of Designing E-Learning Environment based on Mastery Learning and Artificial Intelligence on Developing English Speaking Skills among Tenth Graders in Palestine. Journal of Educational & Psychological Studies, 31(1). https://doi.org/10.33976/IUGJEPS.31.1/2023/22
Alshahrani, A. (2023). The impact of ChatGPT on blended learning: Current trends and future research directions. International Journal of Data and Network Science, 7(4), 2029-2040. https://doi.org/10.5267/j.ijdns.2023.6.010
Alzahrani, A. (2014). The Effects of Instructors’ Technological Pedagogical and Content Knowledge (TPACK) on Online Courses. Doctoral Dissertations. Texas Tech University. http://hdl.handle.net/2346/58720
Azizi, S. M., Roozbahani, N. y Khatony, A. (2020). Factors affecting the acceptance of blended learning in medical education: application of UTAUT2 model. BMC medical education, 20, 1-9. https://doi.org/10.1186/s12909-020-02302-2
Cooper, G. (2023). Examining science education in ChatGPT: An exploratory study of generative artificial intelligence. Journal of Science Education and Technology, 32(3), 444-452. https://doi.org/10.1007/s10956-023-10039-y
Dai, Y., Liu, A. y Lim, C. P. (2023). Reconceptualizing ChatGPT and generative AI as a student-driven innovation in higher education. 33rd CIRP Design Conference, Sydney, Australia. 84-90. https://doi.org/10.1016/j.procir.2023.05.002
Dergaa, I., Chamari, K., Zmijewski, P. y Saad, H. B. (2023). From human writing to artificial intelligence generated text: examining the prospects and potential threats of ChatGPT in academic writing. Biology of Sport, 40(2), 615-622. https://doi.org/10.5114/biolsport.2023.125623
Eysenbach, G. (2023). The role of ChatGPT, generative language models, and artificial intelligence in medical education. JMIR Medical Education, 9(1), 1-13. https://doi.org/10.2196/46885
Foroughi, B., Senali, M. G., Iranmanesh, M., Khanfar, A., Ghobakhloo, M., Annamalai, N. y Naghmeh-Abbaspour, B. (2023). Determinants of intention to use ChatGPT for educational purposes: Findings from PLS-SEM and fsQCA. International Journal of Human-Computer Interaction, 1-20. https://doi.org/10.1080/10447318.2023.2226495
Hair, J., Hollingsworth, C. L., Randolph, A. B. y Chong, A. Y. L. (2017). An updated and expanded assessment of PLS-SEM in information systems research. Industrial Management & Data Systems, 117(3), 442-458. https://doi.org/10.1108/IMDS-04-2016-0130
Henseler, J., Ringle, C. M. y Sarstedt, M. (2015). A new criterion for assessing discriminant validity in variance-based structural equation modeling. Journal of the Academy of Marketing Science, 43, 115-135. https://doi.org/10.1007/s11747-014-0403-8
Islam, I. e Islam, M. N. (2024). Exploring the opportunities and challenges of ChatGPT in academia. Discover Education, 3(1), 31. https://doi.org/10.1007/s44217-024-00114-w
Järvelä, S., Nguyen, A. y Hadwin, A. (2023). Human and artificial intelligence collaboration for socially shared regulation in learning. British Journal of Educational Technology. 54(5), 1057-1087. https://doi.org/10.1111/bjet.13325
Kasneci, E., Seßler, K., Küchemann, S., Bannert, M., Dementieva, D., Fischer, F., Gasser, U., Groh, G., Günnemann, S., Hüllermeier, E., Krusche, S., Kutyniak, G., Michaeli, T., Nerdel, C., Pfeffer, J., Paquet, O., Sailer, M., Schmidt, A., Seidel, T., Stadler, M., ... y Kasneci, G. (2023). ChatGPT for good? On opportunities and challenges of large language models for education. Learning and individual differences, 103. https://doi.org/10.1016/j.lindif.2023.102274
Kopplin, C. S. (2022). Chatbots in the Workplace: A Technology Acceptance Study Applying Uses and Gratifications in Coworking Spaces. Journal of Organizational Computing and Electronic Commerce, 32(3-4), 232-257. https://doi.org/10.1080/10919392.2023.2215666
Li, S., Liu, Y. y Su, Y. S. (2022). Differential analysis of teachers’ technological pedagogical content knowledge (TPACK) abilities according to teaching stages and educational levels. Sustainability, 14(12), 7176. https://doi.org/10.3390/su14127176
Liu, M., Ren, Y., Nyagoga, L. M., Stonier, F., Wu, Z. y Yu, L. (2023). Future of education in the era of generative artificial intelligence: Consensus among Chinese scholars on applications of ChatGPT in schools. Future in Educational Research. 1(1), 72-101. https://doi.org/10.1002/fer3.10
Luo, J. (2024). A critical review of GenAI policies in higher education assessment: A call to reconsider the “originality” of students’ work. Assessment & Evaluation in Higher Education, 1-14. https://doi.org/10.1080/02602938.2024.2309963
Medeiros, M., Ozturk, A., Hancer, M., Weinland, J. y Okumus, B. (2022). Understanding travel tracking mobile application usage: An integration of self determination theory and UTAUT2. Tourism Management Perspectives, 42. https://doi.org/10.1016/j.tmp.2022.100949
Menon, D. y Shilpa, K. (2023). “Chatting with ChatGPT”: Analyzing the factors influencing users' intention to Use the Open AI's ChatGPT using the UTAUT model. Heliyon, 9(11). https://doi.org/10.1016/j.heliyon.2023.e20962
Mohammad-Salehi, B., Vaez-Dalili, M. y Heidari Tabrizi, H. (2021). Investigating Factors That Influence EFL Teachers' Adoption of Web 2.0 Technologies: Evidence from Applying the UTAUT and TPACK. TESL-EJ, 25(1). https://tesl-ej.org/pdf/ej97/a21.pdf
Ning, Y., Zhang, C., Xu, B., Zhou, Y. y Wijaya, T. T. (2024). Teachers’ AI-TPACK: Exploring the relationship between knowledge elements. Sustainability, 16(3), 978. https://doi.org/10.3390/su16030978
Nookhao, S. y Kiattisin, S. (2023). Achieving a successful e-government: Determinants of behavioral intention from Thai citizens’ perspective. Heliyon, 9(8). https://doi.org/10.1016/j.heliyon.2023.e18944
Pavlik, J. V. (2023). Collaborating with ChatGPT: Considering the implications of generative artificial intelligence for journalism and media education. Journalism & Mass Communication Educator, 78(1), 84-93. https://doi.org/10.1177/10776958221149577
Perera, P. y Lankathilaka, M. (2023). Preparing to Revolutionize Education with the Multi-Model GenAI Tool Google Gemini? A Journey towards Effective Policy Making. Journal of Advances in Education and Philosophy, 7(8), 246-253. https://doi.org/10.36348/jaep.2023.v07i08.001
Qin, F., Li, K. y Yan, J. (2020). Understanding user trust in artificial intelligence‐based educational systems: Evidence from China. British Journal of Educational Technology, 51(5), 1693-1710. https://doi.org/10.1111/bjet.12994
Scherer, R., Siddiq, F. y Tondeur, J. (2019). The technology acceptance model (TAM): A meta-analytic structural equation modeling approach to explaining teachers’ adoption of digital technology in education. Computers & Education, 128, 13-35. https://doi.org/10.1016/j.compedu.2018.09.009
Sharples, M. (2023). Towards social generative AI for education: theory, practices and ethics. Learning: Research and Practice, 9(2), 159-167. https://doi.org/10.1080/23735082.2023.2261131
Sidiropoulos, D. y Anagnostopoulos, C. N. (2024). Applications, challenges and ethical issues of AI and ChatGPT in education. arXiv preprint arXiv:2402.07907. https://doi.org/10.48550/arXiv.2402.07907
Smith, A., Hachen, S., Schleifer, R., Bhugra, D., Buadze, A. y Liebrenz, M. (2023). Old dog, new tricks? Exploring the potential functionalities of ChatGPT in supporting educational methods in social psychiatry. International Journal of Social Psychiatry, 69(8), 1882-1889. https://doi.org/10.1177/00207640231178451
Yilmaz, R. y Yilmaz, F. G. K. (2023). Augmented intelligence in programming learning: Examining student views on the use of ChatGPT for programming learning. Computers in Human Behavior: Artificial Humans, 1(2). https://doi.org/10.1016/j.chbah.2023.100005
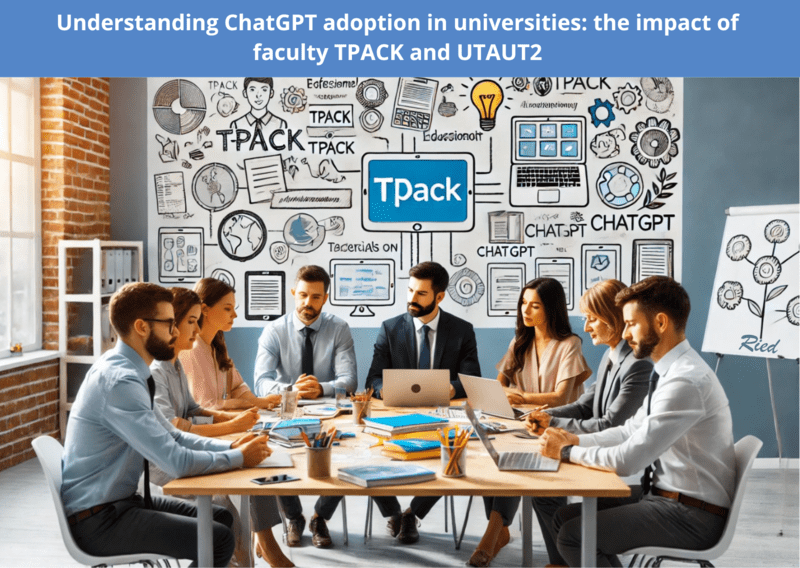